Executive summary
- Generative AI is fundamentally changing content creation, resulting in cost and time savings of 70% and meaningful effectiveness gains
- For today’s business leaders to capitalise on generative AI, executives need to understand the technology and the opportunities it unlocks
- This report provides background on the technology, its business utility and its transformative nature

Foundations
The rise of generative AI
The rise of generative AI seems to have been meteoric. Mentions of Artificial Intelligence in analyst calls have been rising steadily since 2016, but then developments such as OpenAI releasing ChatGPT substantially accelerated that trend.

ChatGPT was initially powered by GPT-3, a Large Language Model (LLM) released in 2020. While the underlying LLM had been publicly available for a year before ChatGPT’s release, the easy user interface made the model accessible to the wider public. This is clear evidence that widespread adoption of a new technology is not only influenced by the sophistication of the AI model, but also by the user experience in which it is made available.
Consumer interest in generative AI also brought new challenges to enterprise leaders. Business leaders had little time to react to employees experimenting with generative AI tools in their daily work. Setting the right AI compliance framework to enable such innovation, while mitigating risk is a business imperative. The work is well under way, with 55% of organisations already piloting generative AI, or have deployed it in production, according to Gartner.

A paradigm shift in creation
What is so transformational about generative AI? Why has there been unprecedented adoption of the technology?
Since 1888, when Kodak sold the first commercial camera, people have been using sensors to capture images and videos. Sensors have greatly improved, going from analogue methods to digital cameras and microphones. Over time, camera sensors became smaller, and with the advent of the internet and smartphones, user generated content (UGC) created a scalable method for content creation in the consumer space.
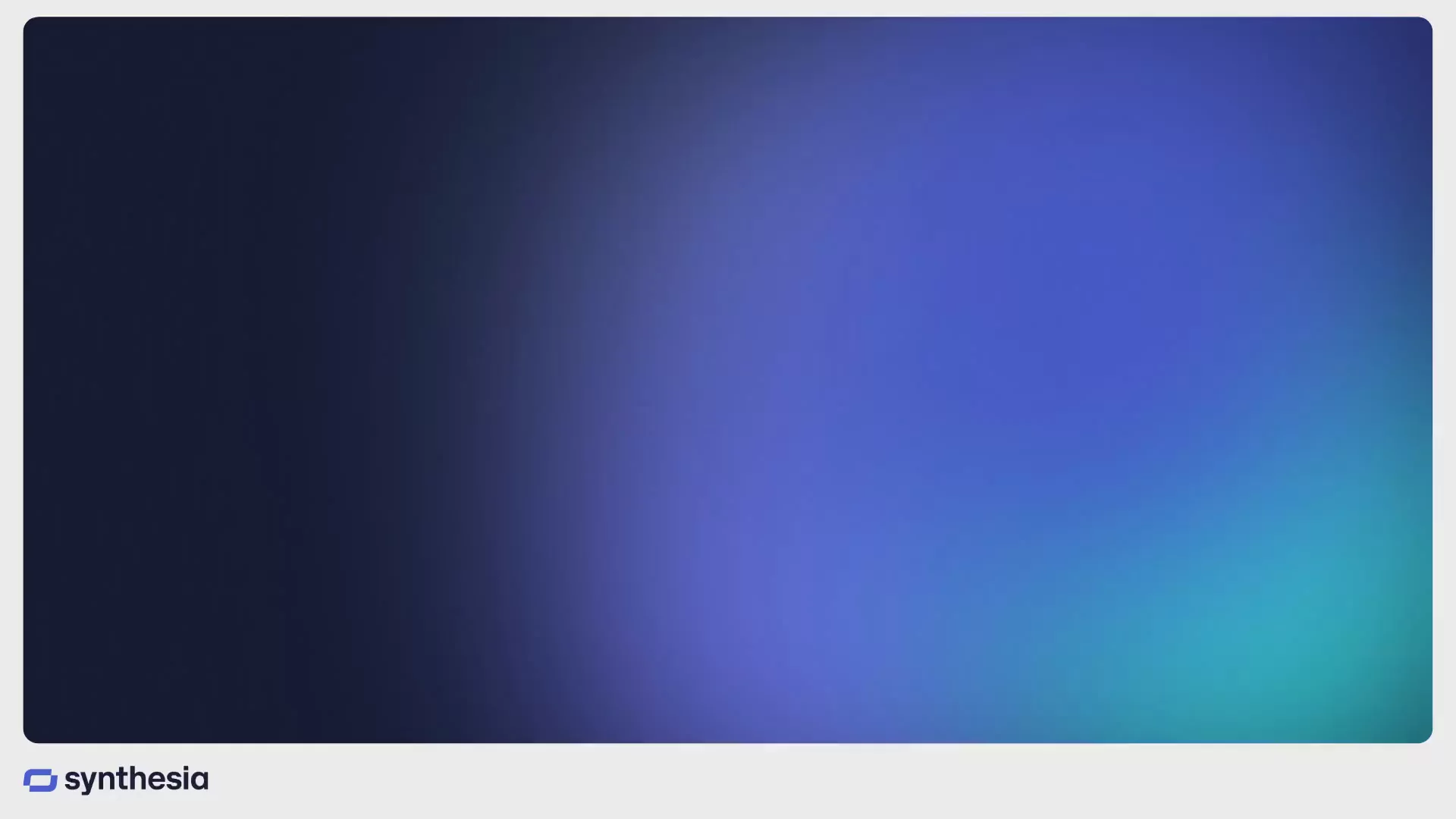
UGC, enabled by mobile, combined with the Internet bringing the marginal cost of distribution to zero, enabled the invention of today’s leading digital platforms, resulting in some of the most lucrative business models ever invented.
But UGC did not meet the content creation needs of the enterprise. Producing content with physical sensors, such as cameras and microphones, is complex and time consuming. These high barriers of entry limited the application of visual formats in the enterprise. Text remained the default format of enterprise communications and knowledge sharing, because text was the only scalable format. Simply put, any employee can type an email or create a presentation.
Generative AI fundamentally changes this, allowing for content to be generated using computers, instead of physical sensors such as cameras or microphones.
Defining generative AI
As such, generative AI can be defined simply as: any text, image, audio or video generated using software, instead of physical sensors like cameras or microphones.
Unlike discriminative AI models that are trained to classify data, generative models undergo a training process to understand the underlying structure and distributions of the training dataset. Once trained, they generate novel outputs that resemble the training data but are not exact replicas.
The supply chain of generative AI
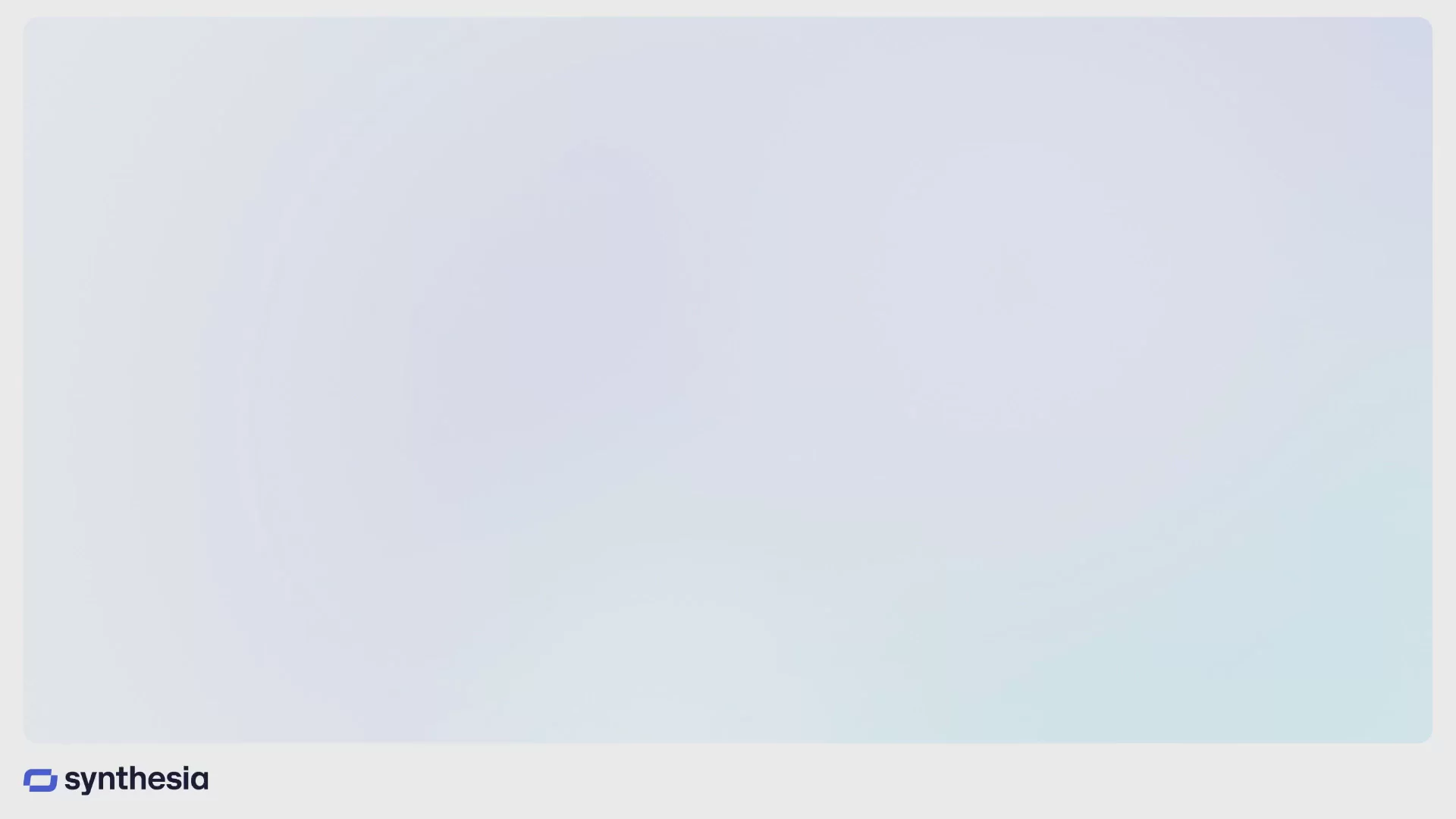
Given its physical nature, the traditional content supply chain is a linear, sequential process.
Now that we can generate content using software, generative AI has transformed the linear supply chain into a vertical supply stack.
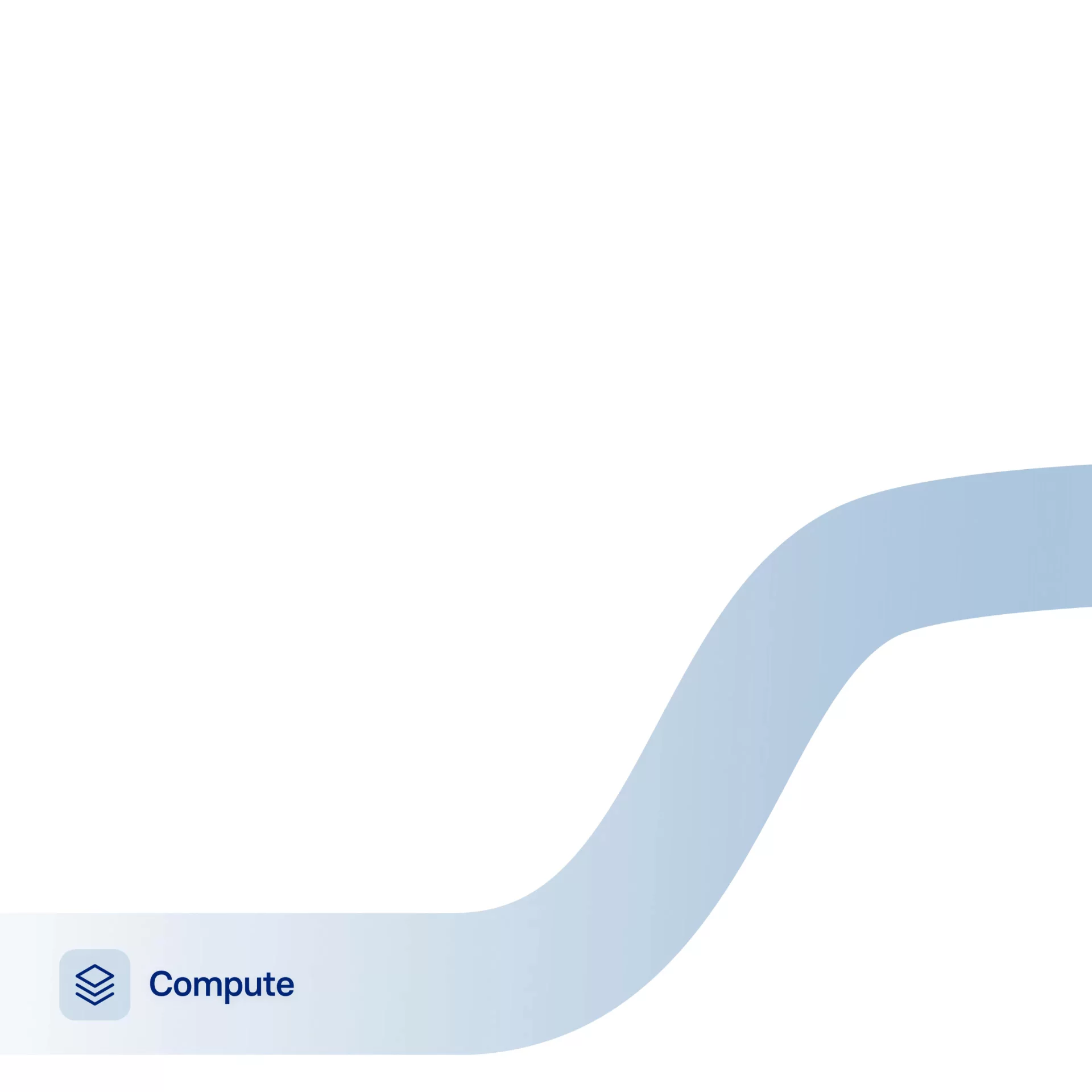
- Services: specialist services to support the deployment of AI models
- Application: B2B and B2C products the end-user interacts with. Domain-specific solutions that solve for a particular user pain point
- Inference: prompting the trained model to generate net new output
- Model governance: maintenance and versioning generative AI models (e.g. fine tuning)
- Training: development of AI models, including data management, model training and evaluation
- Compute: the physical infrastructure used to train, infer and deploy generative AI
Competition in the age of generative AI
Given these changes to the supply chain, businesses need to rethink how to compete in the age of generative AI. One framework to evaluate points of differentiation along each layer of the new supply stack
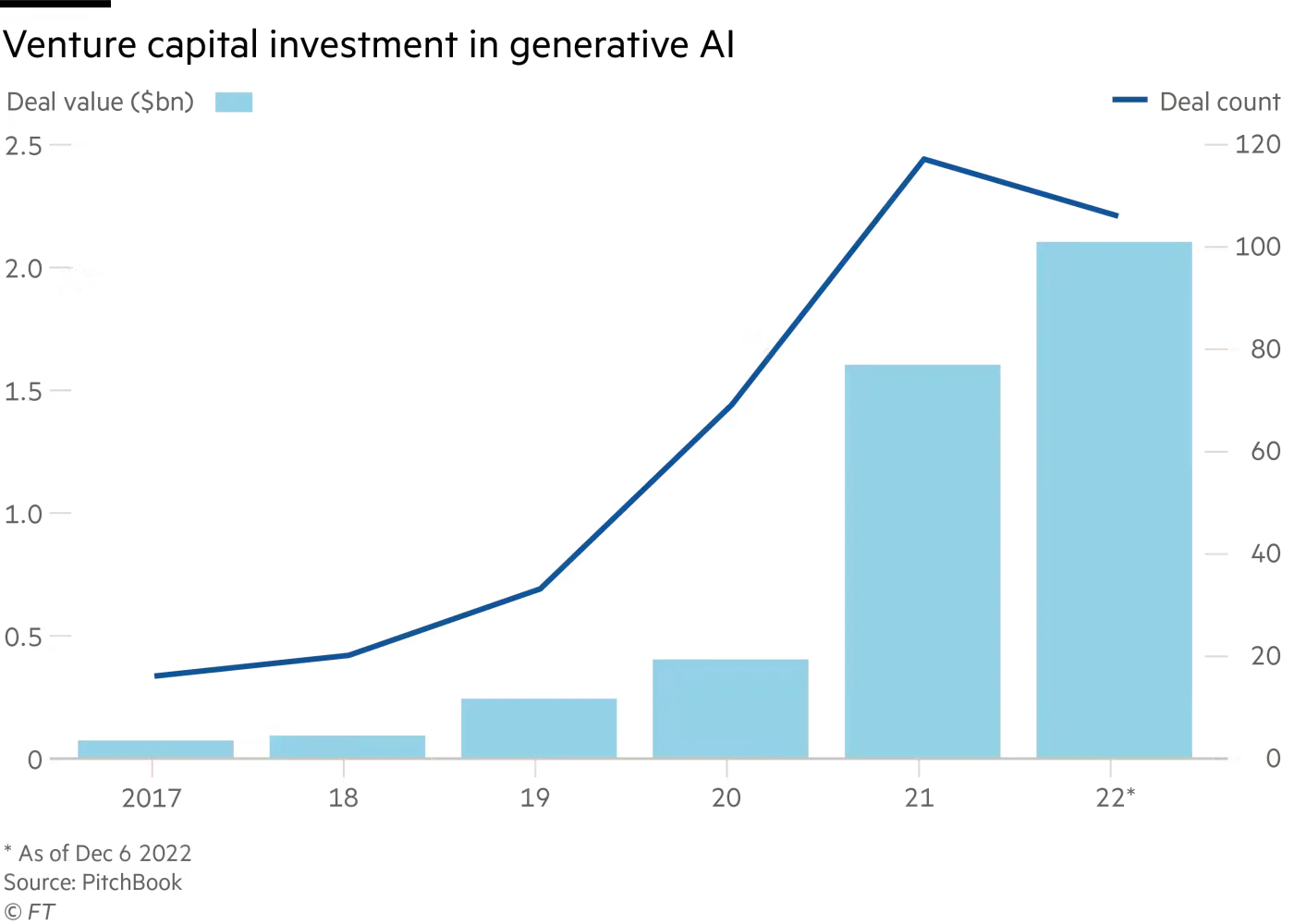
Let’s take Training as an example. The excitement that followed the launch of ChatGPT led to increased investment in generative AI, especially in Large Language Model providers, such as OpenAI or Anthropic.
The increase in capital deployed led higher competition, with both startups and Big Tech racing to develop ever more powerful LLMs.
This race among leading LLM providers has competed away differentiation based on the quality of the text generated. Companies such as OpenAI or google now seek to differentiate at other layers of the supply stack, for example by increasing the context window at the Inference layer.

The LLM Wars also created opportunities for other businesses to differentiate. For example, as competing at the Inference layer intensified, publishers of up-to-date information, such as news outlets or Reddit, began to licence their content to model developers. This created a new revenue stream for publishers and differentiation for the LLM provider by enriching their models with the latest news at Inference.
Similarly, startups can leverage competing LLMs to their advantage, without the CapEx investment required to develop the models. One example is Perplexity, which drives differentiation at the Application layer by offering access to most leading LLMs in its Pro subscription. Bundling leading LLMs into a single $20 monthly subscription drives utility to the end user, who otherwise would need to pay for each LLM separately at triple the cost.

The business utility of generative AI
Big 4 Consultancy doubles conversions with AI video
Early adopters report cost and time savings of 70%, higher engagement from deploying generative AI in the enterprise.
Coding in natural language
Human-like performance in natural language processing has brought on a new era where computers can take human language as input.
This feature reinforces two key aspects of generative AI:
- now that humans can interact with computers without learning to code, barriers to entry are drastically reduced
- now that we can generate content with computers, without physical barriers to production, content gains the scale benefits of software
These two factors reduce both the cost and time required to produce content, while also improving enterprise productivity.
Generative text in the enterprise
Academic research is well underway to quantify the productivity benefits generative AI brings to business. MIT researchers, led by Brynjolfsson, found that introducing generative text into the workflow of 5000 customer support agents increased productivity by 14%.
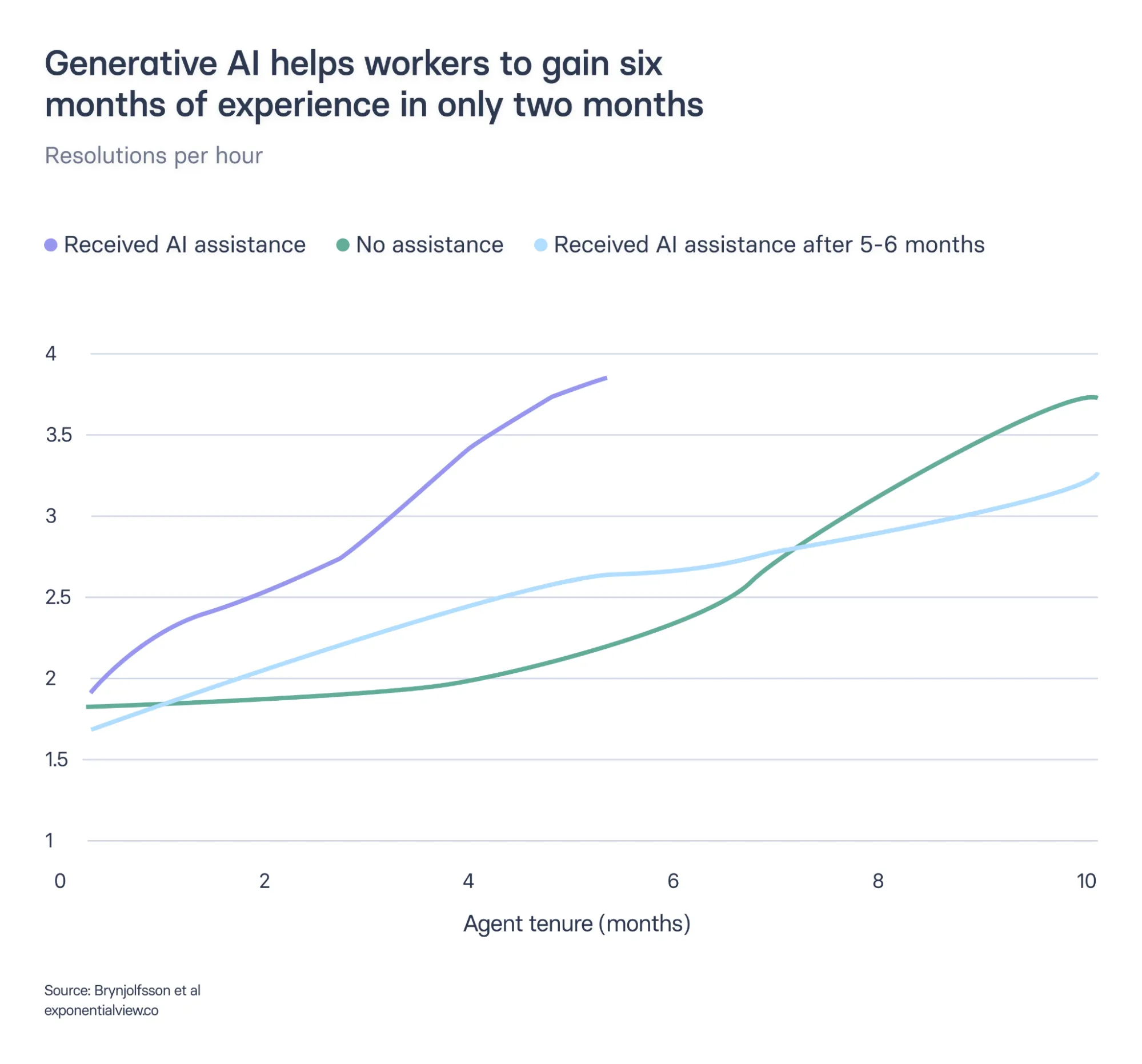
Interestingly enough, the productivity uplift differed based on skill level and experience. With the least experienced employees seeing the biggest productivity uplift (35%), while the best performing employees at the firm experienced no meaningful productivity boost. Researchers also found that generative text enabled faster onboarding of new employees and helped improve poor performance through a continuous feedback loop, rather than periodic managerial reviews.
While no final conclusions can be drawn from a single study, these findings seem to make intuitive sense. Generative models lower the barriers of entry, making it easier and faster for non-experts to create content.
Other working papers from MIT suggest even larger gains, finding that OpenAI’s coding assistant CoPilot increased productivity by 50% for coders who used it to augment their work.
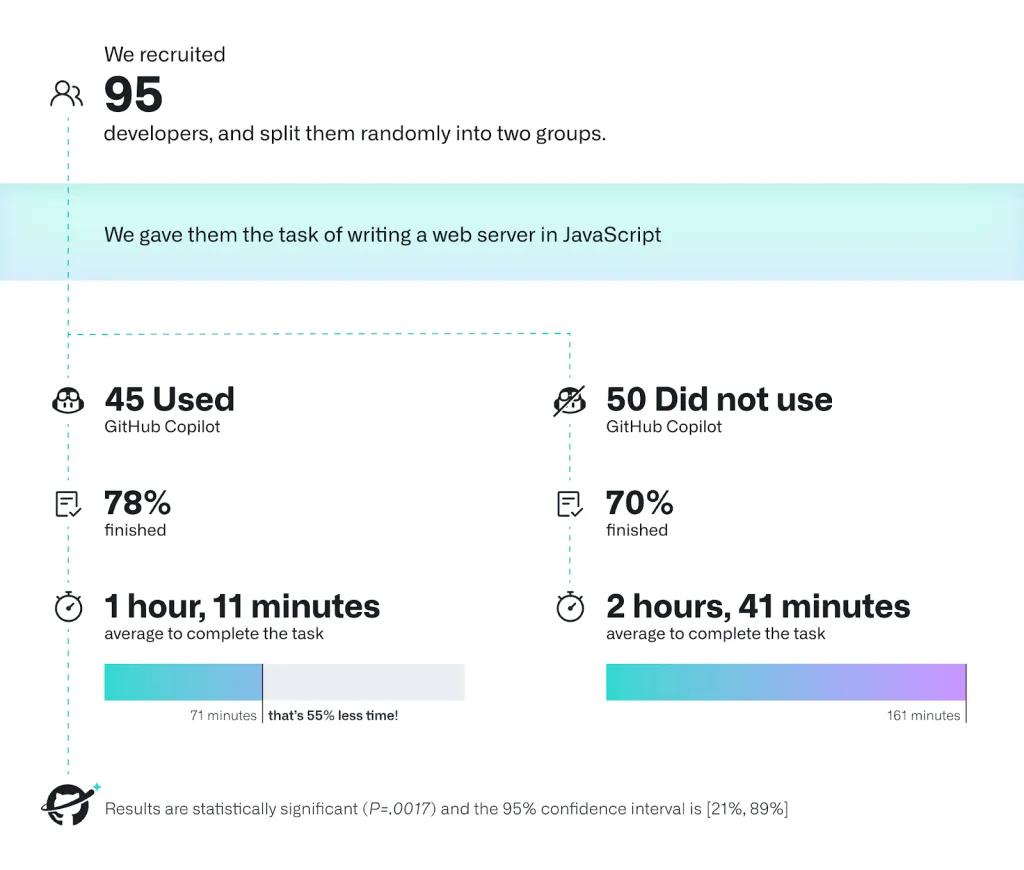
This productivity uplift is meaningfully higher than that observed by Brynjolfsson and team.
Complex production, higher productivity boost

The higher productivity gains might relate to the production complexity of certain formats. While generative text drove efficiency improvements for support agents, text is easier to produce than software code. Industry data suggests that the harder it is to produce a format, the bigger the productivity gain driven by generative AI.
AI video in the enterprise
Traditional video production is a linear process requiring many specialists and equipment to create. From storyboarding, to casting, production and editing, it is a complex and time consuming process. Generative AI transforms the physical process of video production into a digital one, allowing for video to be generated in minutes, rather than planned and produced over weeks. It also reduced the need for costly equipment, explaining the 70-90% efficiency gains reported by enterprise customers. One such example is the enterprise deployment of AI video.
Efficiency gains of 95%
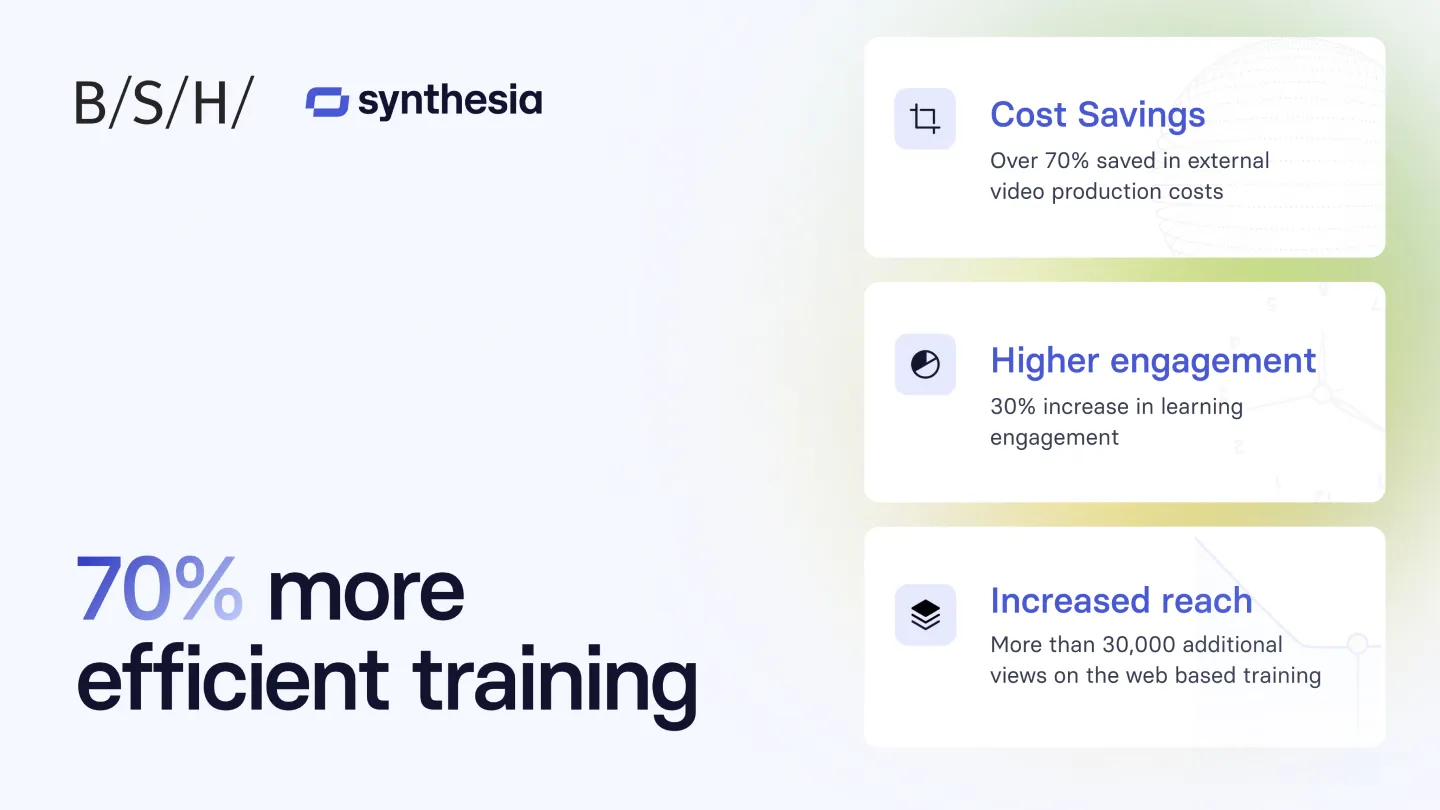
The exponential adoption of AI video is telling of the business value unlocked. For example, the BSH Group reports their team has saved 70% of normal video production cost and time by using generative AI video.
Similar results were observed by Teleperformance, the global customer service giant. Teleperformance reported $5,000 saved per video when generative AI was applied. The UK’s Network Rail saw even higher efficiencies, reporting 95% saved in production time.
The engagement uplift of AI video
Efficiency gains however, are only one aspect of productivity. Effectiveness is just as crucial, whether the enterprise content is meant to educate or to inform.
Replacing text with AI video can increase engagement by 30%, as reported by the BSH Group.
One explanation might be a shift in consumer preference. According to Cisco, 82% of the Internet’s traffic was video in 2022. Video-first platforms such as Tiktok, Instagram and Snapchat, are the most popular iOS apps, according to Business of Apps. Consumer preference for video is clear.
Consumer demand seems to contradict the enterprise’s heavy reliance on text, however. In 2014 alone, 500 billion Word documents were created. The large majority of information and knowledge in the enterprise today is stored in text. While most employees can easily produce text documents or presentations, fewer can design images or produce video with traditional means. Generative AI changes that paradigm, allowing any employee to create video, simply by typing text.
Early research also suggests that AI video is just as effective as its traditional predecessor. Researchers at UCL explored the relationship between the educational value of both AI and traditional video, concluding that there was no meaningful difference in effectiveness.

Enterprise Transformation
AI maturity in the enterprise
While both academia and industry practice show tangible outcomes from generative AI, barriers to adoption remain. While more than half of organisations has adopted the technology, Gartner found that the number 1 challenge remains estimating and demonstrating business value.
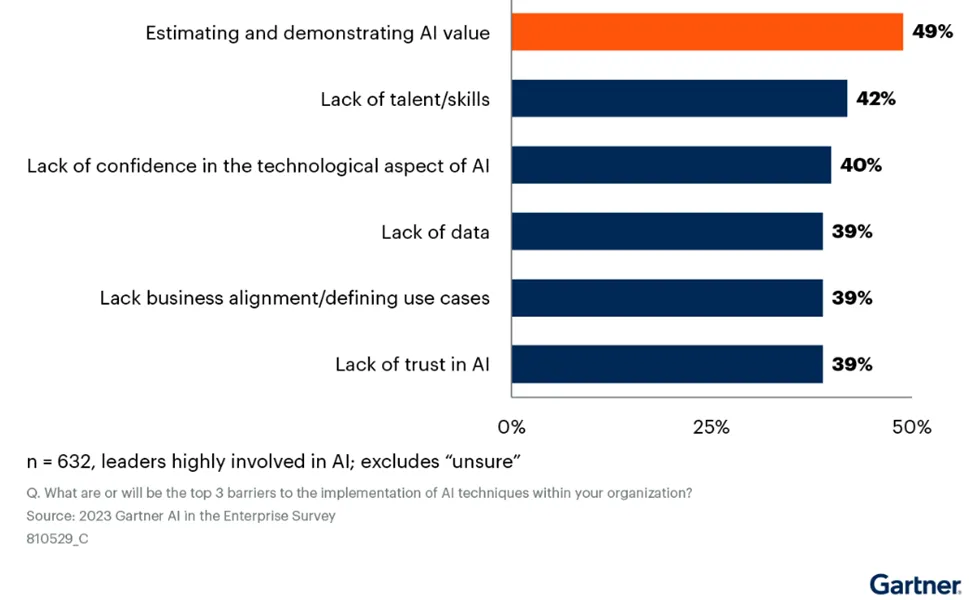
Organisations looking to progress from pilots to production-ready solutions will face mounting pressures to prove real ROI from their technology investment. Two aspects of this shift are transforming the Customer Experience and transforming the Operating Model of the company.
From efficiencies, to top-line growth: transforming CX
Like with any truly transformative technology, generative AI can drive measurable outcomes along two main dimensions:
- Improving the Customer Experience
- Lowering its unit cost
To understand how new technologies can improve the Customer Experience, we can learn from previous transformations.
Over the past decade, user-generated content, combined with the internet, led to new forms of expression and some of the most lucrative business models ever invented.
Over the next decade, AI-generated content will unlock similar results. Those who leverage the features unique to generative AI to improve customer experience will be the ones to see real returns on their investment.
New technologies enable new, native formats that capitalise on features unique to the new platform. When mobile emerged, people didn’t recreate feature films on their phones, but invented mobile-native formats, such as TikTok videos. Transforming the Customer Experience requires AI-native formats that build on features unique to generative AI, such as infinite scalability and low barriers of entry.
The new formats of generative AI
An early example is PepsiCo’s Messi messages campaign, powered by Synthesia, which leverages the unlimited scale of AI video.
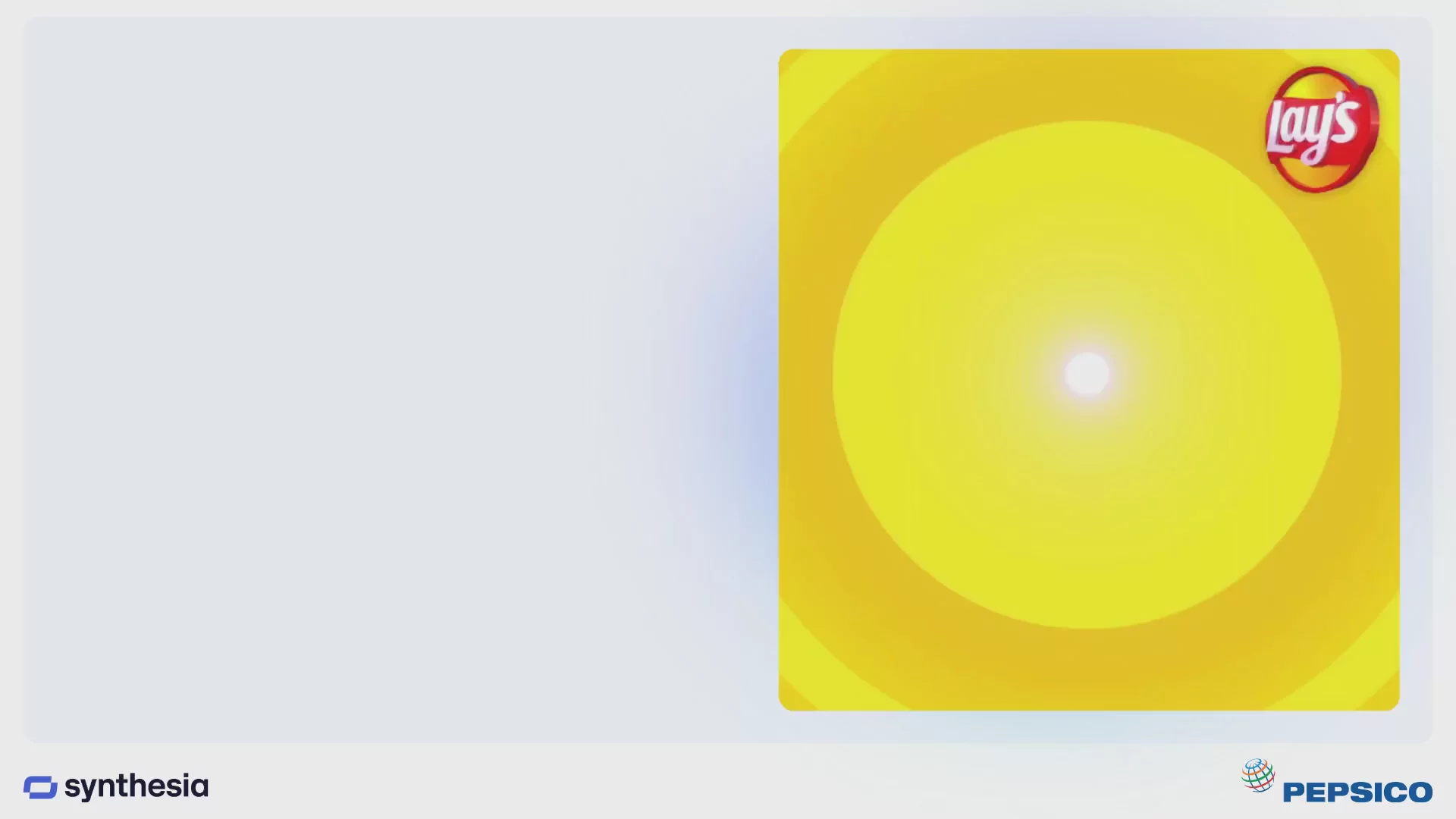
Messi messages allowed for personalised videos to be generated at scale with the football star’s likeness. Fans generated over 7 million videos in eight languages, a feat impossible to achieve with traditional video production.
From customer acquisition, to retention and support, CX needs to be reinvented across the entire life cycle. To achieve this, enterprises must combine multiple tools into an end-to-end solution.
From tools, to solutions: transforming operations
Economic research has shown a lag between the invention of general-purpose technologies and their subsequent impact on productivity growth.
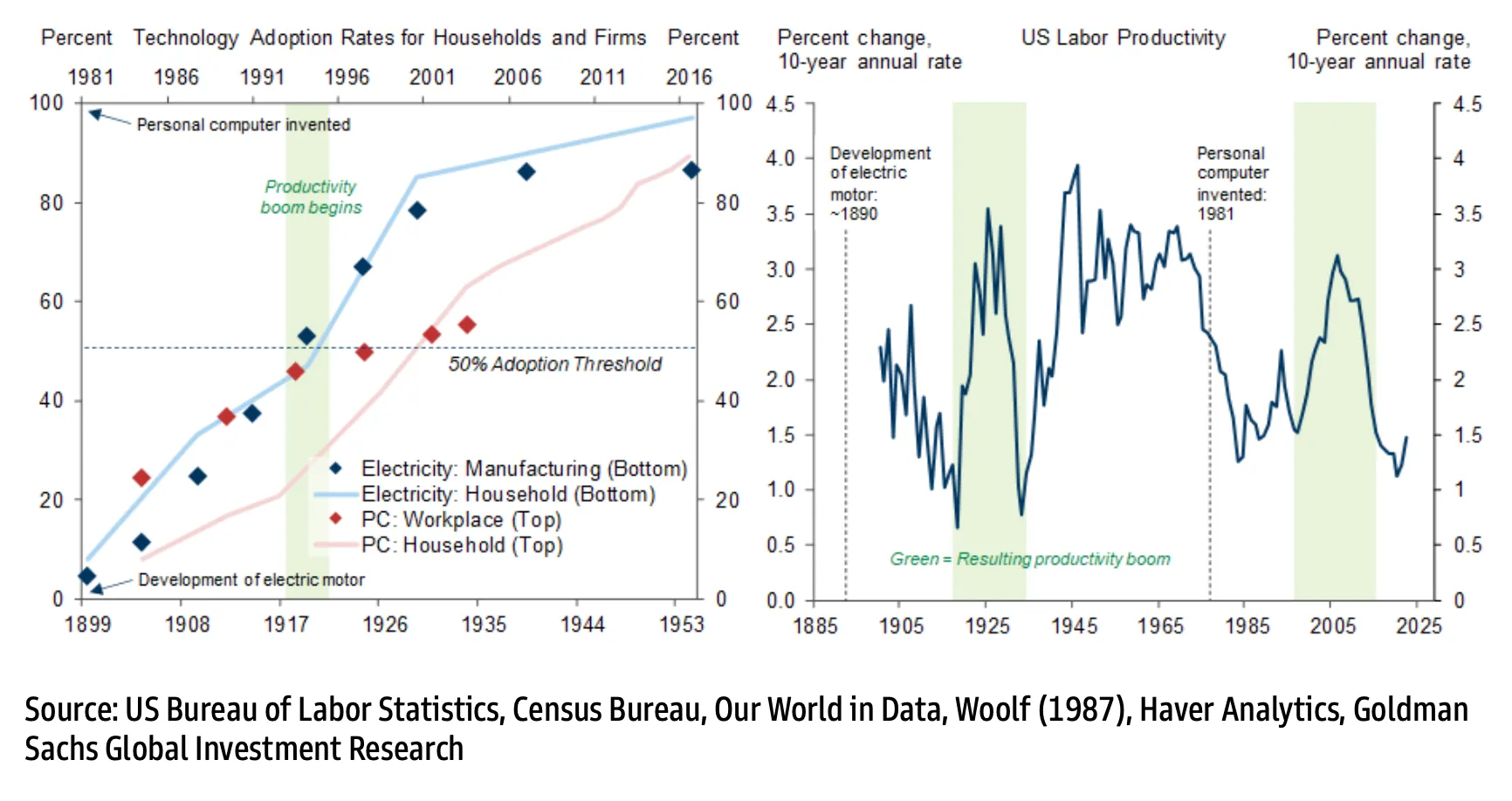
For example, the invention of electricity was without question a pivotal moment in economic development. However, the productivity boom only materialised after factories reorganised around the assembly line.
Sustained value from generative AI should only be expected once the hard work of reinventing business processes and the enterprise operating model has begun.
Reinventing enterprise workflows
Let’s take sales prospecting as an example. Let’s assume our business objective is to increase CRM conversions - and hence drive incremental revenue downstream in the prospecting funnel.
One execution might be to integrate video into prospecting campaigns. While this might prove useful, it also creates additional tasks, increasing the resource requirements of our prospecting efforts.
A better solution might be to re-engineer the prospecting workflow, so that a personalised video generates automatically when a new prospect enters our database. Creating this solution will require up-front investment into tasks such as prompt engineering the brand’s tone into script generation, creating brand compliant video templates for each journey step, or integrating CRM data for a personalised experience. But once the workflow is built, it operates without further manual intervention, reducing the marginal cost of the system.
Transforming the enterprise operating model
Creating these solutions is only half of the equation. Just as important is to reinvent the operating model of the enterprise. The assembly line didn’t simply change the physical layout of a factory, but also changed how humans operate within. From training to safety policies, it required the hardest transformation to implement: changing human behaviour.
Let’s take content localisation as an example. Currently, most international companies use a hub-and-spoke model to localise content, with meaningful operational overhead stemming from global-local team collaboration. Additional challenges pile on if the company works with external agencies, easily adding weeks to completion.
Augmented by AI translation, organisations need to rethink the operating model from the ground up. Central roles might shift from coordinating stakeholders to more hand-on execution. Many of the tasks delegated to agencies might be in-housed cost effectively. These changes are hard to implement on a global scale, with transformation programmes taking years to complete.
As such, the full potential of generative AI might take decades to materialise. Native formats are yet to be invented, enterprise workflows to be built and enterprise operations need to be reoriented.
Some evidence suggests however that adoption might be more seamless than previous transitions. As generative AI systems develop better reasoning capabilities, human-machine collaboration becomes more effective.
As Professor Brynjolfsson notes:
“Often with new technologies, there’s a bit of a decline before it takes off, because it’s difficult and costly to implement changes, retrain workers, and change business processes. In this case, we did not see a lull, and performance took off over just a few months.”
About the author
Synthesia is a leading AI video platform for the enterprise, serving over 55,000 clients globally, including half of the Fortune 100.
Daniel Verten
Daniel leads Synthesia’s Strategy function, working closely with the AI video creation platform’s Fortune 100 clients. In his role, Daniel supports business leaders as they navigate the complex landscape of AI transformation. He has made it his mission to ensure generative AI drives tangible business outcomes and it is applied responsibly in the enterprise.
Sources:
https://www.tker.co/p/nvidia-earnings-q1-2024-ai-demand-boom
https://arxiv.org/abs/2304.11771
https://arxiv.org/pdf/2302.06590.pdf, https://economics.mit.edu/sites/default/files/inline-files/Noy_Zhang_1.pdf
https://www.synthesia.io/case-studies
https://www.synthesia.io/case-studies/bosch
https://www.synthesia.io/case-studies/teleperformance
https://www.synthesia.io/case-studies/network-rail
https://www.synthesia.io/case-studies/bosch
https://www.businessofapps.com/data/most-popular-apps/
https://www.businessinsider.com/how-many-microsoft-office-documents-were-made-last-year-2014-2
https://arxiv.org/abs/2304.03784
Generative AI Can Boost Productivity Without Replacing Workers